What are monitoring systems for land-based greenhouse gas fluxes?
Carbon monitoring systems provide annual data on greenhouse gas (GHG) emissions and sequestration (collectively, “fluxes”) to measure progress toward decarbonization, inform policymaking, and manage regulatory or incentive programs. However, land-based GHG sources and sinks pose challenges for accurate monitoring. Unlike energy-based GHG sources, GHG fluxes from land uses like forests, agriculture, and wetlands arise from both natural and anthropogenic processes that vary across the landscape and over time. These fluxes must therefore be estimated from sample measurements and remotely sensed data, or from proxy data like land management records paired with carbon models.
How do monitoring systems for land-based greenhouse gas fluxes work?
Robust monitoring systems include several complementary components to address the complexity and variability of land-based GHG fluxes:
- Sampling networks provide repeated measurements of attributes linked to carbon stocks, like tree diameter, canopy height, and soil bulk density.
- Remote sensing tools like satellite imagery provide spatial data on land cover, which is necessary to extrapolate sample measurements to a landscape scale. More advanced remote sensing tools like lidar and 3D photography can also be calibrated with sample measurements to provide high-resolution maps of carbon stocks in trees and forests.
- Practice monitoring through remote sensing, sample-based observation, or landowner surveys provides data on key land management practices, like agricultural tillage and cropping systems. These data are necessary inputs into carbon models to estimate agricultural GHG fluxes.
- Carbon models translate sample measurements and management practice information into estimates of GHG flux in soils. GHG flux estimates for trees and forests are more simply calculated using equations derived from scientific studies.
Each of these monitoring system components can introduce uncertainty into the final estimate of GHG flux. This means that the total uncertainty in these estimates can be quite large, limiting the application of monitoring data for policies like market mechanisms or incentive programs that aim to increase land-based carbon removal. There are three primary strategies to expand the usefulness of monitoring systems by reducing this uncertainty:
- Make data more timely by collecting updated data regularly and minimizing the “lag” time between data collection and publication. Timely data on GHG fluxes can help ensure that policies and investments are translating to GHG outcomes as expected—and if they are not, timely data can inform real-time course corrections.
- Expand the scope of data collection by taking repeated sample measurements of land-based carbon pools that currently have limited field data available, like agricultural soils, urban trees, and wetlands. Instituting sample-based monitoring systems for these carbon pools could improve the accuracy of estimates for total land-based GHG flux.
- Enhance the resolution of monitoring datasets by collecting more sample measurements and/or utilizing advanced remote sensing tools. Monitoring systems with a finer spatial resolution (the minimum area size for which GHG fluxes can be measured) are more useful for downscaling to state and local GHG inventories, or even managing individual fields or forest stands. Monitoring systems with a finer temporal resolution (the minimum length of time over which fluxes can be measured) are better able to isolate specific causes of GHG flux—for example, separating the effects of wildfire from timber harvesting—which can make them more useful for targeted policymaking and land management.
Key design considerations
What methods will the monitoring system use to collect data and estimate GHG fluxes? Will the methodologies adhere to standardized protocols (e.g., guidelines from the Intergovernmental Panel on Climate Change)? Who will verify the accuracy of data outputs from the monitoring system?
What level of uncertainty is acceptable for estimates of land-based GHG flux? What tools (e.g., adding more sample plots or integrating lidar data) will the monitoring system use to increase precision?
How frequently will monitoring data be updated? How quickly after data collection will the data be published?
What land uses will the monitoring system cover?
Will the monitoring system be spatially explicit (i.e., mapped to locations) or rely on statistical estimation? What is the minimum area size (e.g., 1 square meter or county-level) for which data will be available? What is the minimum length of time (e.g., month, year, or multi-year) for which data will be available?
Will the monitoring system include projections for planning? How will future data be estimated?
What financial investments, labor requirements, or agency capacities are required to administer the monitoring system? What is the return on investment (e.g., reduced uncertainty, greater timeliness or enhanced spatial resolution) for new monitoring methods or tools?
U.S. experience with monitoring systems for land-based greenhouse gas fluxes
U.S. estimates of GHG fluxes in forests are derived from the Forest Inventory and Analysis (FIA) program, administered by the USDA Forest Service. FIA uses satellite imagery to identify forested areas across the United States and surveys a network of field plots to collect data on forest type, tree size, and other forest attributes. Each field plot is remeasured every 5-10 years, with measurements averaged across space and time to produce annualized estimates of GHG flux at the national and state level. FIA officials are exploring ways to improve the program’s data resolution and coverage of trees outside forests, but may require greater federal support to make these improvements.
U.S. estimates of GHG fluxes on agricultural lands are derived from the National Resources Inventory (NRI) and the DayCent carbon model. NRI, administered by the Natural Resources Conservation Service (NRCS), collects data on soil, water, and land management every three years from a network of field plots across all nonfederal lands. The DayCent model from Colorado State University estimates GHG fluxes annually as a function of environmental conditions and the most recent NRI data. Deeper integration of remote sensing tools and incorporation of soil carbon measurement on a subset of NRI plots could improve the timeliness and accuracy of GHG flux estimates on agricultural lands.
Other federal datasets contribute relevant information for estimating land-based GHG fluxes, but have various drawbacks that limit their utility for more robust monitoring applications. They include:
- National Land Cover Database (NLCD)—provides comprehensive geospatial data on land cover across the United States, but not timely due to five-year remeasurement cycle and three-year time lag before data are released
- Cropland Effects Assessment Program (CEAP)—provides more granular data on agricultural soil management practices but has not been updated regularly, limiting its value for regular reporting requirements like the National GHG Inventory
- National Wetlands Inventory (NWI)—maps wetlands across the United States but does not collect data on wetland management, which is essential for GHG estimation
- Resource Planning Act (RPA) Assessment—assesses and projects baseline trends in forest and grassland resources on a ten-year cycle, but excludes croplands and other land uses
Current estimates of land-based GHG fluxes based on these data sources are highly uncertain, contributing over 70% of the total margin of error in the economy-wide National GHG Inventory. However, some states have been taking steps to reduce this uncertainty in their own monitoring systems. For example, doubling the number of sample plots in California led to a 30% decrease in the margin of error in its forest inventory. In Minnesota, lidar-assisted models improved the precision of forest stocking estimates tenfold over federal inventory methods.
resources on a ten-year cycle, but excludes croplands and other land uses
Additional Resources
- ClimateWorks Foundation, “Reducing climate policy risk: improving certainty and accuracy in the U.S. land use, land use change, and forestry greenhouse gas inventory,” 2019, https://www.climateworks.org/wp-content/uploads/2019/09/Reducing-Climate-Policy-Risk-REPORT.pdf
- National Academies of Sciences, “Terrestrial carbon removal and sequestration,” 2018, in Negative Emissions Technologies and Reliable Sequestration: A Research Agenda, https://www.nap.edu/read/25259/chapter/5
- United States Environmental Protection Agency, “Inventory of U.S. greenhouse gas emissions and sinks, 1990-2018,” 2020, https://www.epa.gov/sites/production/files/2020-04/documents/us-ghg-inventory-2020-main-text.pdf
- World Resources Institute, “CarbonShot: Federal Policy Options for Carbon Removal in the United States,” 2020, https://www.wri.org/publication/carbonshot-federal-policy-options-for-carbon-removal-in-the-united-states
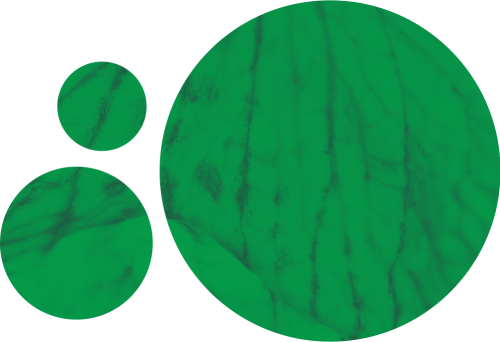